In the latest round of MLPerf Inference – a suite of standardized, peer-reviewed inference benchmarks – the NVIDIA platform delivered outstanding performance across the board. Among the many submissions made using the NVIDIA platform were results using the NVIDIA GH200 Grace Hopper Superchip. GH200 tightly couples an NVIDIA Grace CPU with an NVIDIA Hopper GPU using NVIDIA NVLink-C2C, a high-bandwidth, low-latency interconnect for superchips.
In this post, we take a closer look at the great performance demonstrated by servers powered by the NVIDIA GH200 in the latest round of MLPerf Inference benchmarks.
The NVIDIA GH200 Grace Hopper Superchip is a new type of converged CPU and GPU architecture combining the high-performance and power efficient NVIDIA Grace CPU with the powerful Hopper GPU using NVLink-C2C, delivering 900 GB/s of bandwidth to the GPU, 7x faster than todays’ servers. With GH200, the CPU and GPU share a single per-process page table, enabling all CPU and GPU threads to access all system-allocated memory that can reside on physical CPU or GPU memory. When adopted, this architecture removes the need to copy memory back and forth between the CPU and GPU.
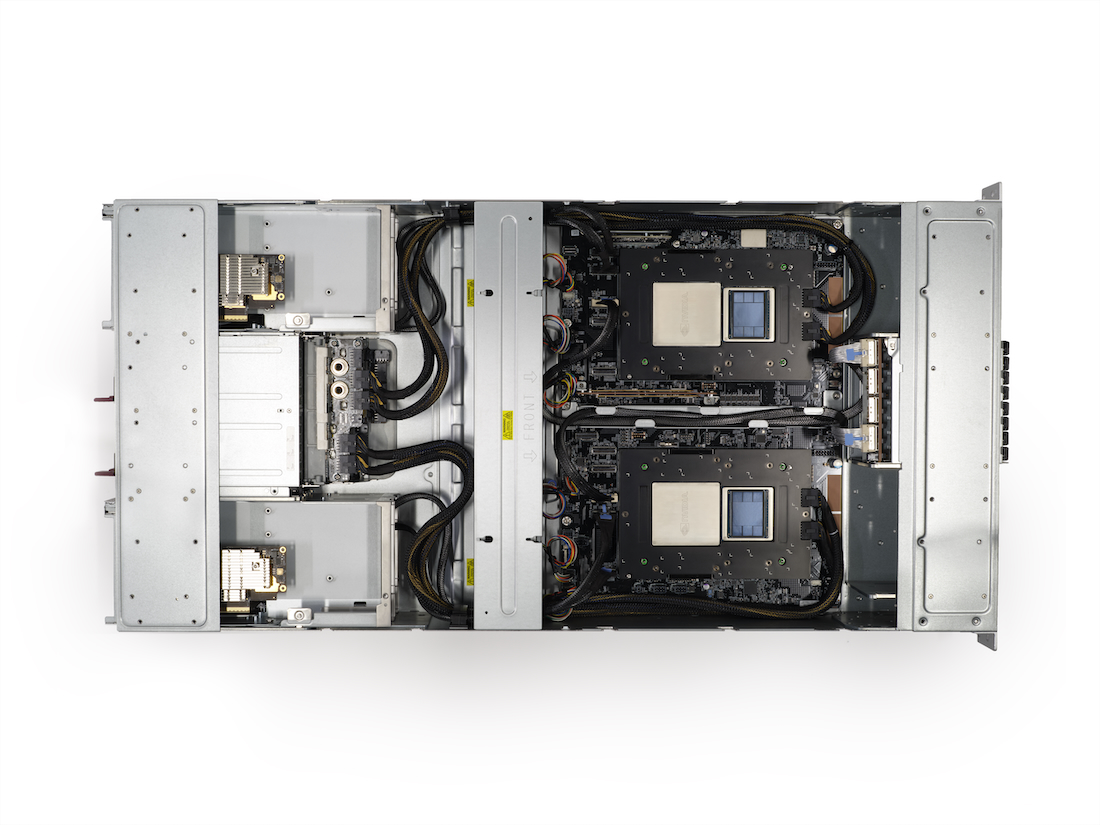
NVIDIA GH200 NVL2 builds on the successes of the NVIDIA GH200 by connecting two GH200 Superchips with NVLink in a single node, making it easier to deploy, manage, and scale to meet the demands of single-node LLM inference, Retrieval Augmented Generation (RAG), recommenders, graph neural networks (GNNs), high-performance computing (HPC) and data processing.
The GH200 NVL2 fuses two Grace CPUs and two Hopper GPUs in an innovative architecture delivering 8 petaflops of AI performance into a single node. The Grace CPUs come with 144 Arm Neoverse cores and up to 960GB of LPDDR5X memory. The Hopper GPUs offer 288GB of the latest HBM3e memory and up to 10TB/s of memory bandwidth, 3.5x and 3x more than the H100 GPU respectively. This simplifies development with the coherent memory, delivers leading performance in a single server and allows customers to scale out to meet demand.
GH200 delivers world-class generative AI performance
On a per-accelerator basis, the NVIDIA GH200 delivered outstanding inference performance across every generative AI benchmark in MLPerf Inference v4.1. Across the two most demanding LLM benchmarks – Mixtral 8x7B and Llama 2 70B – as well as DLRMv2, representing recommender systems, GH200 delivered up to 1.4x more performance per accelerator compared to the H100 Tensor Core GPU.
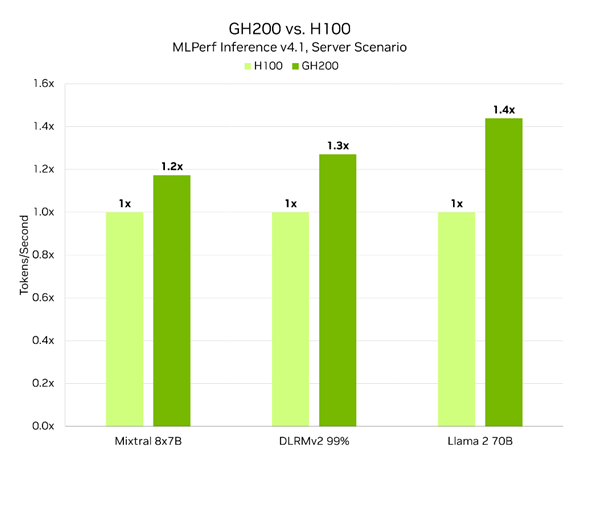
And, compared to the best two-socket, CPU-only submissions using currently-available x86 CPUs, a single GH200 Grace Hopper Superchip delivered up to 22x higher throughput on the GPT-J benchmark. There were no CPU-only submissions on the more challenging Llama 2 70B or Mixtral 8x7B benchmarks.
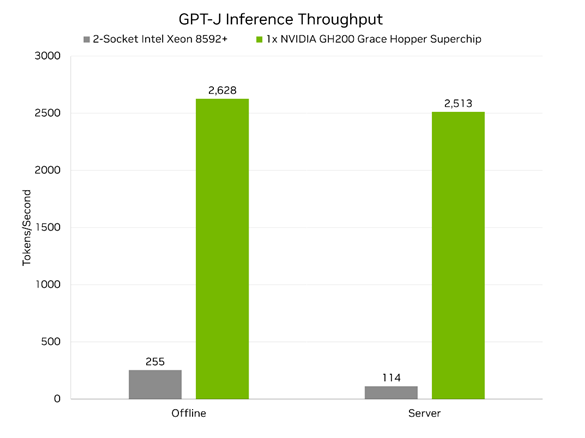
Additionally, many organizations looking to deploy generative AI workloads in production are looking to run real-time, user-facing services. The MLPerf Inference server scenario aims to measure inference throughput under defined latency constraints, better representing popular real-time use cases than the offline scenario.
Also note that while GH200 delivers server scenario performance within 5% of its offline performance, on the challenging Llama 2 70B benchmark, the best CPU-only submission using x86 sees performance degrade by 55% in the server scenario compared to the offline scenario.
While many small- and mid-size generative AI models and use cases – including all MLPerf Inference v4.1 benchmarks – can run optimally on a single GH200 Superchip, there are scenarios where multiple accelerators need to work in tandem to meet latency constraints. For example, while Llama 3.1 70B can fit comfortably in the memory of a single Hopper GPU, deployments with more stringent latency requirements benefit from the combined AI compute performance provided by the two Hopper GPUs connected via NVLink in the GH200 NVL2.
The ecosystem is embracing NVIDIA GH200
In this round of MLPerf Inference, many NVIDIA partners made submissions using their NVIDIA GH200 and GH200 NVL2-based server designs, including server makers Hewlett-Packard Enterprise (HPE) that submitted one GH200 NVL2-based design, QCT and Supermicro, as well as cloud service provider Oracle Cloud Infrastructure (OCI).
HPE:
“We are already seeing outstanding performance with the HPE ProLiant Compute DL384 Gen12 with a submission based on the NVIDIA GH200 NVL2 design in no small part due to the 144GB HBM3e memory available per Superchip,” said Kenneth Leach, Principal AI Performance Engineer and MLCommons representative at HPE. “As part of the NVIDIA AI Computing by HPE portfolio, we’ve proven time and again that this platform can deliver high performance for generative AI inference, and appreciate NVIDIA’s continued collaboration. As the first company to submit performance results with this version of the NVIDIA GH200 NVL2, HPE is incredibly proud of the work we continue to pioneer through our long-standing NVIDIA partnership.”
Oracle:
“We validated the combination of Grace CPU and H200 GPU, connected with NVLink interconnect, for AI inference. This demonstrated the outstanding performance of this NVIDIA architecture, and the even greater potential of the upcoming Grace Blackwell. We look forward to supporting customers with the OCI Supercluster based on NVIDIA Grace Blackwell Superchips,” said Sanjay Basu, Senior Director, Cloud Engineering, Oracle Cloud Infrastructure.
QCT:
“With the GH200’s groundbreaking architecture, we’re equipped to enhance developer productivity and drive the next wave of AI applications. Our MLPerf results highlight GH200’s potential to bring AI into enterprise systems to meet the computational demands of modern data centers,” said Mike Yang, Executive Vice President of Quanta Computer Inc. and President of QCT.
Supermicro:
“To help data centers manage rising power demands from AI workloads, the GH200 offers exceptional efficiency. In addition, our MLPerf test submission showcases the significant performance increase that GH200 unlocks for customers.”
Conclusion
The GH200 demonstrated great performance and versatility in the latest MLPerf Inference benchmarks. GH200 NVL2, available today, provides up to 3.5x more GPU memory capacity and 3x more bandwidth than the H100 for compute- and memory-intensive workloads. With a balanced CPU-GPU architecture to address a wide variety of enterprise needs, a flexible 2U air-cooled MGX platform design that’s easy to deploy and scale out, and a 1.2 TB pool of fast memory, it is an ideal solution for enterprises looking to run mainstream LLMs and the large and expanding universe of CUDA-accelerated applications.
Oracle Future Product Disclaimer
The preceding is intended to outline Oracle’s general product direction. It is intended for information purposes only, and may not be incorporated into any contract. It is not a commitment to deliver any material, code, or functionality, and should not be relied upon in making purchasing decisions. The development, release, timing, and pricing of any features or functionality described for Oracle’s products may change and remains at the sole discretion of Oracle Corporation.