Reality capture creates highly accurate, detailed, and immersive digital representations of environments. Innovations in site scanning and accelerated data processing, and emerging technologies like neural radiance fields (NeRFs) and Gaussian splatting are significantly enhancing the capabilities of reality capture. These technologies are revolutionizing interactions with and analyses of the physical world.Â
Site scanning, the first step of reality capture, generates detailed 3D models using methods like Lidar and photogrammetry, while accelerated processing, powered by NVIDIA RTX GPUs, enable faster and more efficient data handling. NeRFs excel in producing photorealistic 3D scenes, and Gaussian splatting offers a novel approach to smooth, efficient rendering. AI enhances these tools by providing deeper insights through advanced algorithms for object detection, segmentation, and classification.Â
This post explores how NVIDIA is at the forefront of the integration of AI with reality capture, driving these technological advancements with powerful GPUs, software solutions, and cutting-edge research.
Reality capture basics
The reality capture process begins with scanning or photographing a physical environment, which is then processed through photogrammetry or Lidar to generate a point cloud—a dense collection of data points that represent precise 3D surface locations. This point cloud is often converted into a 3D model, providing a detailed virtual representation of the physical space.
Photogrammetry
Photogrammetry is a technique that uses photographic images to extract detailed spatial information about physical objects, including their distances, dimensions, shapes, and exact positions in space. By analyzing angles, overlaps, and perspectives from multiple viewpoints, photogrammetry can create point clouds, which are then converted to highly detailed 3D models.Â
This method is accessible and cost-effective, particularly when compared to Lidar, as it only requires basic photographic equipment. However, the accuracy of photogrammetry is highly dependent on the quality and quantity of the images captured, and it can struggle with certain surfaces like those that are reflective or transparent, which can result in less reliable outcomes.
Lidar
Lidar (light detection and ranging) technology uses laser pulses to measure distances and create precise 3D models of environments by calculating the time it takes for light to reflect back from surfaces. It offers unparalleled accuracy in capturing detailed spatial data over large areas, even in challenging lighting conditions (such as low light or darkness), and is effective at mapping various materials, including vegetation and surfaces beneath transparent objects.Â
Lidar is typically more expensive than photogrammetry due to the specialized hardware required, and it can struggle with highly reflective surfaces like water or glass, potentially distorting data or creating gaps. Additionally, while Lidar excels in geometric accuracy, it provides less texture information compared to photogrammetry, which can limit its effectiveness in applications requiring photorealistic detail.
Point clouds and 3D meshes
Point clouds and 3D meshes are essential elements of reality capture, converting raw data from Lidar or photogrammetry into detailed, accurate virtual models. Point clouds consist of dense collections of points mapping precise 3D surface locations, which are often converted into 3D meshes that form continuous, textured surfaces for more realistic representations.Â
CUDA, NVIDIA RTX, and the NVIDIA Omniverse platform significantly enhance this process. CUDA accelerates the complex computation needed for processing large datasets, RTX enables real-time ray-traced rendering for highly realistic lighting and shadows, and Omniverse provides a powerful collaborative platform for editing and visualizing 3D meshes seamlessly in real time.Â
Choosing the right reality capture technology
Choosing the right reality capture technology depends on your project’s specific needs. Lidar is the go-to for high-resolution, detailed spatial data, making it ideal for large-scale surveying, complex sites, and environments where precision is paramount. Software like Autodesk ReCap and Bentley iTwin Capture are commonly used to streamline Lidar data processing and analysis.Â
Photogrammetry, on the other hand, excels in capturing detailed color data, which is particularly useful in architectural documentation and cultural heritage preservation. Drones equipped with high-resolution cameras can significantly enhance photogrammetry by capturing images from multiple angles and difficult-to-reach areas, enabling comprehensive 3D models of large or complex sites. Tools like Esri Site Scan for ArcGIS and Pix4D are widely used in photogrammetry, providing robust solutions for processing drone-captured imagery into detailed 3D models.
Enhancing workflows with CUDA and NVIDIA RTX
To handle the massive datasets that are typically involved with reality capture, CUDA accelerates the processing of Lidar point clouds and photogrammetric data by leveraging parallel computing, significantly reducing the time required for data conversion, visualization, and analysis. This makes it valuable for high-resolution scanning and 3D reconstruction projects.
RTX technology enhances the visualization of these 3D models by incorporating ray tracing, which delivers photorealistic lighting, shadows, and reflections. This capability is crucial for creating immersive, high-fidelity visualizations of scanned environments, with tools like Omniverse and Unreal Engine offering RTX-powered rendering for both Lidar and photogrammetry workflows.
NeRFs and Gaussian splatting
NeRFs are transforming 3D scene synthesis by using machine learning to generate highly detailed and realistic views from a vastly reduced number of 2D images compared to traditional photogrammetry. NeRFs can interpolate between sparse data points, creating smooth, photorealistic scenes even from angles that weren’t originally captured.Â
This ability to work with fewer images while still delivering exceptional visual fidelity makes NeRFs ideal for applications like architectural visualizations and virtual reality environments. Tools like NeRF Studio enhance the capabilities of NeRFs by enabling developers to add functionalities like semantic embeddings, allowing for more advanced applications and richer interactive experiences.Â
Despite their efficiency, NeRFs still require significant computational resources and high-quality images to function effectively, which can limit their practicality for real-time processing or dynamic environments. NVIDIA is advancing NeRF technology with research projects like NVIDIA NeRF-XL for large-scale models and NVIDIA Instant-NeRF for accelerated processing, pushing the limits of what’s possible in reality capture.
Gaussian splatting is a highly efficient technique for real-time rendering of 3D surfaces or volumes by laying out 2D splats—small, overlapping Gaussian functions—in 2.5D space, enabling smooth, continuous visualizations that balance detail and performance. It excels in scenarios requiring fast and clear visualization of complex 3D point clouds, making it ideal for applications in construction, urban planning, augmented reality, and virtual reality.Â
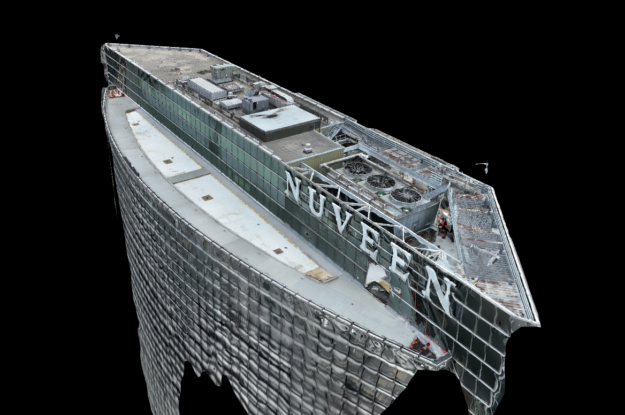
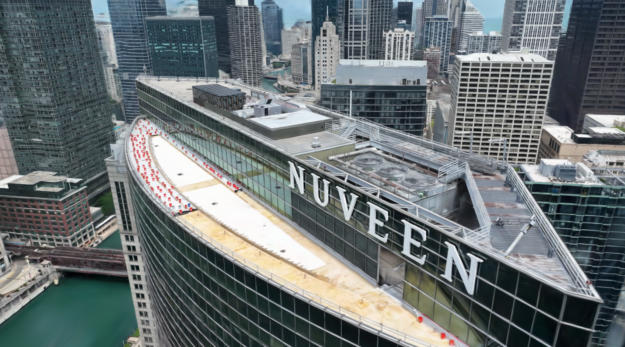
Figure 1. Photogrammetry captures the structure’s meshes but misses the surroundings and background (left). Gaussian splatting includes the background, providing better visualization for developers and use cases that benefit from full context (right). Photo credit: Ben Stocker, Skender
While it provides exceptional smoothness and speed, there is a trade-off in geometric accuracy, which may limit its use in contexts requiring high fidelity. NVIDIA has advanced this technology with tools like NVIDIA InstantSplat for rapid 3D reconstruction, NVIDIA 4D-Rotor Gaussian splatting for real-time dynamic scene visualization, and NVIDIA Align Your Gaussians (AYG) for generating high-quality 4D visualizations from text descriptions. Supported by the Omniverse platform, these innovations enable efficient, detailed, and real-time visualizations in large-scale projects and dynamic environments, offering significant benefits for architectural visualization, construction monitoring, and digital content creation.Â
Startups like Atomic Maps are pushing the boundaries by integrating Gaussian splats into Cesium tiles, providing map-level geographic context that enhances visualization with a comprehensive geographic framework.
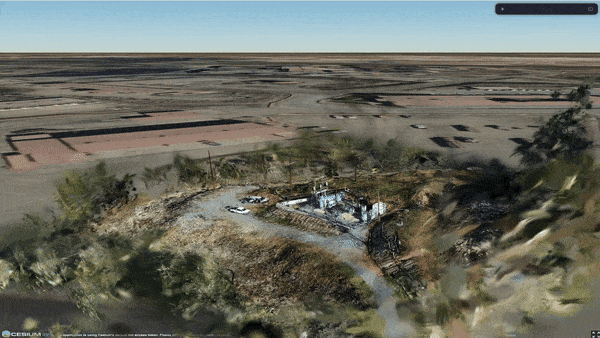
These technologies enable more accurate and immersive representations of environments by capturing intricate details and contextual elements that traditional photogrammetry may miss. While photogrammetry excels in precise measurements and surveying, NeRFs and Gaussian splatting offer superior visual fidelity, enabling developers, building owners, and stakeholders to visualize projects with rich background context, such as cityscape views from a building’s balcony, and to see fine details like phone lines and traffic signs that are often absent in standard photogrammetry. These enhanced visualizations provide a more comprehensive understanding of a project, helping to inform better decisions during the design, planning, and construction phases.
AI for reality capture
AI is transforming reality capture by significantly improving object identification, segmentation, and 3D reconstruction processes. Startups like Hover are leading the charge in using AI to generate detailed 3D models of buildings, enhancing the accuracy and efficiency of structural analysis and categorization.Â
NVIDIA Research is advancing segmentation, a critical aspect of reality capture, with the SAL (segment anything in Lidar) method, which uses a text-promptable, zero-shot model to segment and classify objects in Lidar data without manual supervision. This streamlines workflows and enables more flexible and scalable segmentation. Tools like Gauzilla further expand these capabilities by introducing spatial time lapses, which help visualize structural changes over time, offering deeper insights into project development and maintenance needs.
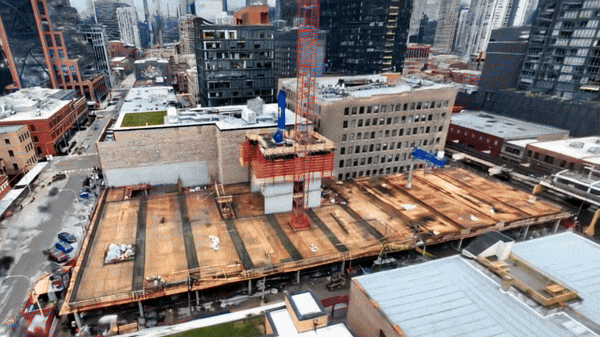
Companies are increasingly using AI and autonomous robotics to streamline reality capture processes. Field AI Field Foundation Models (FFMs) enable autonomous robots to operate in complex, GPS-denied environments, capturing high-quality reality capture data that can be integrated with platforms like Naska.AI through an open partnership model. Naska.AI then uses this data to automate the comparison of laser scans with building information modeling (BIM), highlighting critical information early to reduce costs and prevent schedule overruns, ultimately improving construction accuracy and efficiency.
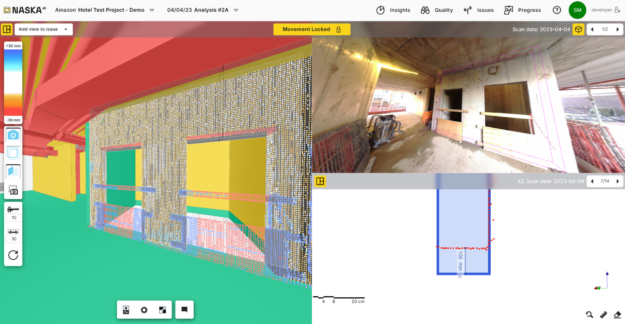
NVIDIA is further advancing reality capture with fVDB, which transforms NeRF and Lidar data into large-scale, AI-ready environments in real time, ideal for urban planning, autonomous systems, and digital twins. Neuralangelo, an AI model from NVIDIA Research, converts 2D video into detailed 3D structures with intricate textures, supporting applications in art, video games, and industrial digital twins.
Conclusion
NVIDIA development tools enable software developers to significantly accelerate reality capture workflows and embed AI pipelines for object identification, segmentation, classification, and 3D reconstruction. These innovations streamline processes, improve accuracy, and expand the potential of reality capture. With NVIDIA RTX GPU acceleration powered by CUDA, enterprises can now process and visualize reality capture data faster and with greater precision, pushing the boundaries of what’s possible in architecture and urban development.
Explore more NVIDIA solutions for the AEC industry.Â
Acknowledgments
Francis Williams, Senior Researcher, NVIDIA
Zan Gojcic, Senior Researcher, NVIDIA
Michael Rubloff, Founder and Managing Editor, Radiancefields.com
Jonathan Stephens, Chief Evangelist and Marketing Director, EveryPoint
Ben Stocker, Senior Construction Technologist, Skender
Michal Gula, Chief Technology Officer, Overhead4D
Chantal Matar, Founder, Studio Chantal Matar
Jim Young, Managing Director, Atomic Maps
Stuart Maggs, CEO, Co Founder Naska.ai